Up until fairly recently, the financial industry has been regarded as a slow mammoth seemingly resisting innovation forever but this has completely changed in recent years and Fintech (financial technology) seems to be one of the most exciting “trends” affecting everything from investments and loans to simple online purchases.
If we look into the numbers on Statista we can see that there are more than 25 000 startups in the fintech space this year alone and their number is growing steadily. Investment value in the field was more than 247 billion U.S. dollars in 2021 but slowed a bit down in the last two years but I would argue that this is just the natural aftermath of the technology bubble effect created by COVID-19.
I don’t think that the barrier of entry ever was low in the financial field, dealing with money is always attractive and brings in many players, you always feel that you can make the most money where the money is flowing but naturally, this also results in really strict regulations. It is no surprise when we look at RegData’s Industry Regulation Index and find that Finance and insurance, transportation, and manufacturing remain the most regulated industries in the U.S. on a federal level. On top of that, industry reports from the likes of Fintech Global (confirmed by my own personal experiences recently) show that due to the increasing number of financial frauds, the financial sector has become even more cautious in letting new players in so the bar is higher than it ever was before.
So, what kind of regulations protect us and our money?
Obviously, you can’t just decide to open a bank out of the blue, the moment you are dealing with people’s hard-earned money regulators step in and apply their protective measures. We are lucky to have worked on a number of European fintech projects before and we are right in the middle of releasing a new fintech product in the US so I can say with firsthand experience that although the EU and USA markets are in theory really similar, they can still be quite different in terms of the regulations and requirements when new digital products enter the market.
More than 40% of financial institutions report a year-on-year increase in fraudulent activities and in fighting that there’s a rapid adoption of Artificial Intelligence (AI) and Machine Learning (ML) technologies to combat financial fraud. A recent study suggests that compared to only 34% in 2022, now 66% of financial institutions are either in the process of implementing or already using AI and ML-powered systems.
To protect consumers, investors, and the integrity of the financial markets fraud prevention is subject to a variety of legal requirements and regulations in the US. The most important of these are worth noting:
- Securities and Exchange Commission (SEC): The SEC is responsible for regulating securities and investment markets. It enforces various laws, such as the Securities Act of 1933 and the Securities Exchange Act of 1934, to ensure transparency and fairness in the financial industry.
- Anti-Money Laundering (AML) Laws: The Bank Secrecy Act (BSA) and the USA PATRIOT Act require financial institutions to have robust AML programs to prevent money laundering and terrorist financing. These laws mandate the reporting of suspicious transactions and the verification of customer identities.
- Know Your Customer (KYC): Financial institutions are required to establish and maintain customer identification programs (CIPs) as part of their KYC procedures. This is essential for verifying the identities of customers and identifying potential risks.
- Dodd-Frank Wall Street Reform and Consumer Protection Act: This act introduced significant reforms to the financial industry in response to the 2008 financial crisis. It created the Consumer Financial Protection Bureau (CFPB) to protect consumers and implemented various regulations to enhance market transparency and prevent fraudulent practices.
- Fair Credit Reporting Act (FCRA): The FCRA regulates the collection, dissemination, and use of consumer information, including credit reports. It ensures the accuracy and privacy of consumer data, which is essential in preventing identity theft and fraud.
- Sarbanes-Oxley Act: Enacted in the wake of corporate accounting scandals such as Enron, this law established stricter corporate governance and financial reporting standards for publicly traded companies. It includes requirements related to internal controls and the certification of financial statements.
- Whistleblower Protections: Various federal laws, such as the Dodd-Frank Act, provide protections and incentives for whistleblowers who report securities fraud and other financial misconduct to the SEC.
- Payment Card Industry Data Security Standard (PCI DSS): For organizations that handle credit card payments, compliance with PCI DSS is required to safeguard cardholder data and prevent payment card fraud.
- Federal Trade Commission (FTC) Act: The FTC enforces consumer protection laws and regulations, including those related to deceptive advertising, online privacy, and the prevention of fraud.
- State Laws: Each state may have its own specific laws and regulations related to financial fraud and consumer protection but these can vary widely.
However, it’s important to add that financial fraud prevention and compliance requirements can vary depending on the type of financial institution, the services offered, and the specific activities involved. You must take these seriously and as a company operating in the financial sector, you are expected to adhere to these laws and regulations to prevent and address financial fraud. Non-compliance can result in civil and criminal penalties, including fines, legal actions or even imprisonment.
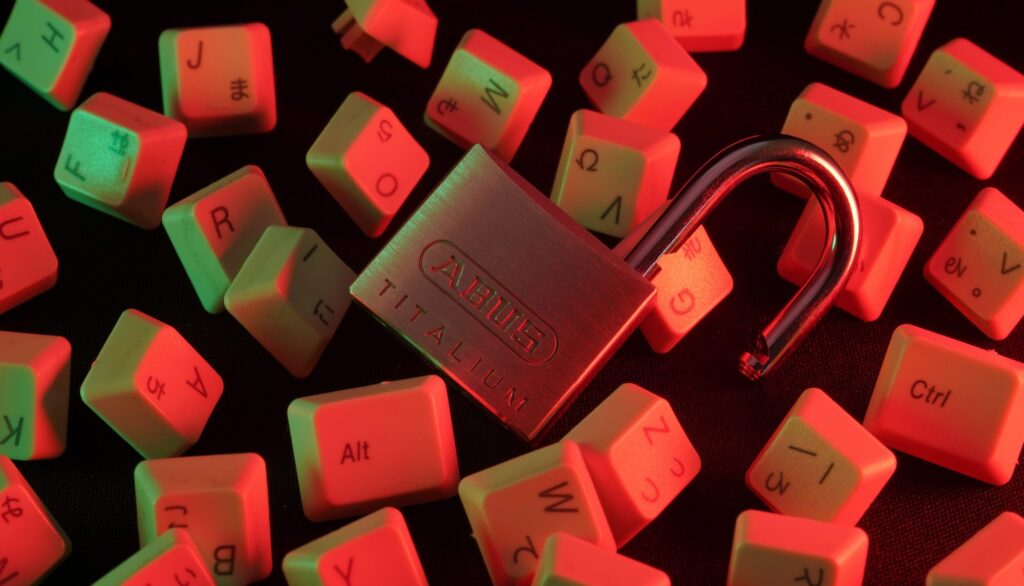
How does AI come into the picture?
Fintech loves the 3 letter acronyms, KYC (know your customer), AML (anti-money laundering), and CIP (customer identification program) are all there in the background from the very first step you register in a new financial app or go into a bank to take a loan. What AI is really good at is looking at historical data and trying to find patterns, learn from them, and prevent the same things from happening again in the future. AI-based fraud detection systems can monitor incoming data to try and minimize fraud threats but based on previously collected (user, transaction, fraud.. etc.) data it can also adjust its behavior to stop threats it may never have seen before and this is what makes it stand out in comparison to traditional rule-based fraud prevention systems.
To give you a simple example, when registering to any new neobank solution (think Revolut and like), you need to enter your personal information, validate your email address and phone number, enter your ID card details, and possibly show your face to the camera (much like in modern airports). In the background, there are a number of checks trying to validate your identity against government registry records, checking if you are indeed a live person and you are not under arrest… etc. These systems usually provide a risk score resulting in a multi-factor risk matrix that would be impossible to check real-time if you would be doing it manually.
The most common types of fraud that can be detected with AI
Let me start with that AI is most effective when it is used as part of a layered security approach that combines multiple tools and strategies. Attack techniques constantly evolve so consequently your AI stack also needs constant updates and adjustments.
While AI can greatly enhance security, it should not be your single line of defense, and companies should also focus on creating adequate policies (security, data management and storage..etc.), internal training and best practices to strengthen their overall cybersecurity.
- Fake Account Creation
Account creation is already a tedious process and usually a painful churning point so this is where most users just drop it if you make the registration process too complicated. However, it is enough to give a quick check on Facebook and you will immediately find a number of fake accounts created by bots, and such automated bots can create fake accounts at incredible speeds but AI can come in handy as it can track many variables to block bots without changing the account creation process.
- Account Takeover (ATO)
The sibling of fake account creation, account takeover is equally dangerous and can also ruin your company’s reputation quickly. ATOs are apparently on the rise as 55% of e-commerce merchants reported an increase in ATO attacks compared to previous years.
Multi-factor authentication is usually a good way to prevent ATOs but many users just don’t enable it.
- Card Fraud
Fraudsters use bots to crack cards, often via brute force attacks that can severely strain payment gateways. Card fraud is one of the most common types of fraud, with a predicted increase in fraudulent transactions growing to $38.5 billion in 2027 from $32.04 billion in 2021.
AI monitors user behavior to distinguish bots from people and block malicious activity or validate the identity of the user and doesn’t just rely on IPs and IP reputation to stop incoming threats.
- Credential Stuffing
During credential stuffing, an automation tries to input common usernames and passwords collected from previous data breaches combined with simple or reused passwords and enters it into your login page. This results in a surprisingly high success rate that can not only crash your login page but it can also lead to ATOs or carding.
Luckily modern AI solutions can track changes in website traffic, a higher-than-usual login failure rate, and other variables to determine if you’re under a credential-stuffing attack.
- Transaction-level Fraud Prevention
AI can also be used to assess transaction data, looking for inconsistencies or patterns that might indicate fraudulent activities. For example, it can identify large, unusual transactions or multiple small transactions that can be grouped and linked to suspicious activity.
- Phishing Attacks
We can analyze emails and messages with AI tools to identify phishing attempts. These can recognize patterns and content or wording that is typical of phishing messages and can help filter out or flag any suspicious messaging.
This is usually done by employing techniques like Anomaly Detection, User Behavior Analysis, or even Voice Analysis. With the help of AI, we can continuously monitor and analyze network traffic, user behavior, and transaction data and detect unusual patterns or deviations from the normal baselines. We can also create behavior profiles to be able to immediately detect deviations or anomalies that may suggest unauthorized access or fraudulent activity.

How is AI used on the other side of the fence?
Of course, it is an endless cat-and-mouse game and attackers are also employing the state of the art AI and ML tools to help their harmful cause. My friend often tells the story about one of his customers developing various laser-based systems and how this particular research lab is visited yearly by companies developing police equipment and also companies developing laser blocking systems against the very same traffipax systems using basically the same technology and it is only a matter of who has the latest iteration and can get ahead a tiny bit.
- Phishing Attacks
AI-powered chatbots can simulate real-time conversations with victims, making phishing attacks more successful. They can generate convincing and personalized phishing emails or messages by analyzing the writing style of legitimate senders and crafting deceptive messages that are more successful in deceiving recipients.
- Credential Stuffing
By using machine learning algorithms to analyze stolen or leaked usernames and passwords and trying to match them with various online accounts AI can adapt to different login forms and security measures making it much more successful in credential-stuffing attacks.
- Identity Theft
By gathering and aggregating personal information from multiple sources like social media profiles, public records, and other online data AI tools can piece together synthetic identities or commit identity theft.
- Fraudulent Transactions
AI can automate the process of conducting fraudulent financial transactions. It can analyze transaction data to identify vulnerabilities and weaknesses in payment systems and execute large-scale transactions without triggering alarms.
- Trading Algorithms and Market Manipulation
In the financial context, algorithms can exploit market inefficiencies, execute high-frequency trading strategies, and manipulate asset prices to drive markets in a certain direction.
- Voice Synthesis
Deepfake voice synthesis technology can be used to impersonate individuals over the phone, creating lifelike voice recordings to facilitate social engineering attacks.
- Bypassing Security Measures
AI can continuously update its methods to evade detection, making it more challenging for security systems to keep up with emerging threats.
- Fraud Detection Evasion
In a reverse application, AI can be used to analyze fraud detection systems and identify their patterns and weaknesses. This can be used to fine-tune fraudulent activities to evade detection.
As you can see AI is a double-edged sword in cybersecurity. It can be used by malicious actors to enhance the effectiveness of financial bot attacks as much as it is also used by cybersecurity professionals to develop more advanced threat detection and prevention systems.
Let’s see the Pros and Cons
As with every technology, there are major pros and cons when looking into AI tools. The best-of-breed financial AI tools are not only dynamic and can adapt quickly but can also process incoming data and block any new threats in milliseconds providing real-time detection. Also, usually (at least to a certain point) more data equals better performance so their performance gets better over time especially if you have high-quality data and if AI instances can share their knowledge. Such tools also reduce the need for human intervention and free up staff to concentrate on other important aspects of your business.
On the other hand, however, AI is known to create so-called false positives. High-quality solutions can minimize this risk but it is currently impossible to eliminate this entirely. AI will occasionally block some real users for example going through a VPN or choosing some esoteric browsers. When you combine AI with ML and neural networks that almost simulate a person’s brain it is quite hard to understand how it actually works or predict how it will react. Good tools give you plenty of customizability but in the background, the system will still very much feel like a black box. Last but not least you still can’t really combat human error and it only takes one employee falling for a phishing attack so you can’t skip continuously educating your team to fight for example social fraud and social engineering types of attacks.
AI and ML are brilliantly exciting fields and this conversation about their ethical and not-so-ethical use could go on forever so if you fancy to join the chat, just drop us a line here and we will be more than happy to give a few tips and advices.